Daily news shows the growing impact and threat of Unmanned Aerial Vehicle (UAV). In modern warfare or for public safety, they extend the possibilities of intelligence or terrorism. All over the world, major efforts are being made to set up anti-drone systems. Acoustic detection is one of the options available to enhance current multimodal detection systems. This paper reviews the state of the art in this field and presents possible techniques for spatial separation of acoustic signals to improve the signal-to-noise ratio, as well as possible classification methods such as machine learning or artificial intelligence.
UAV Detection from Acoustic Signature: Requirements and State of the Art
UAV Detection from Acoustic Signature: Requirements and State of the Art
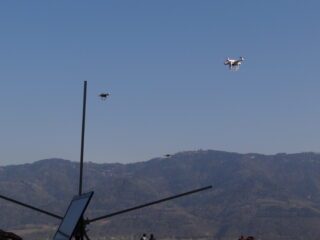
Authors
Lucille Pinel Lamotte, Valentin Baron, Simon Bouley
MicrodB
28 chemin du Petit Bois, 69130 Ecully, France
Abstract
The detection, identification and classification of micro-Unmanned Aerial Vehicles (UAVs) using their acoustic signature is at an early stage where the current performances do not meet the market requirements. They are targeted to complete electro-optical and radio frequency sensors acting in short distances (between 200 and 500 meters). This study firstly defines the detailed requirements to develop effective and affordable countermeasures to report of UAV flying over critical areas, especially in urban areas. It concerns Signal-to-Noise Ratio (SNR) required by the environment and UAV type/distance, the operational frequency domain with the best SNR, the localization accuracy required for neutralization, the real time capabilities to act as soon as possible…
The sound landscape observation is a complex task which has to isolate the noise sources of interest in short delay. The second part of the study establishes a state of the art of the available technologies on the market and the academic works addressing this topic and details how the current solutions cover the complete procedure from detection to classification. For the detection, the system tries to measure the acoustic signature using a single microphone or more complex sensors for a better directivity. For the identification and classification, a Machine Learning procedure is well-suited to recognize UAV audio fingerprint. For now, many academic papers demonstrate the low maturity of the procedure and the need to improve its reliability.
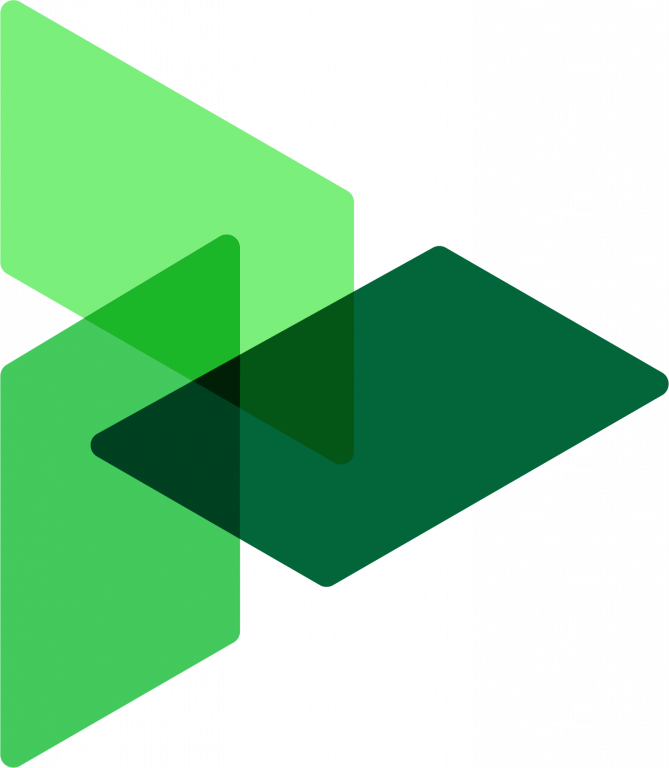
UAV Detection from Acoustic Signature: Requirements and State of the Art
Consult the full publication